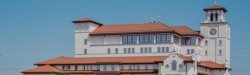
University Calendar
Distantly-supervised Language Technologies for Social Text Analysis

Tuesday October 26, 4-5pm
Anjalie Field, Carnegie Mellon University/University of Washington
Distantly-supervised Language Technologies for Social Text Analysis
https://montclair.zoom.us/j/83405870395?pwd=Y1AwMlA1aEpNK1U1WmZMNUROWU9GQT09
Recent years have seen rapid advances in natural language processing (NLP), especially for supervised tasks. However, it remains infeasible to collect annotated data for supervised training in many settings: social-oriented tasks like detecting bias or stereotypes are difficult for human annotators, and the rapid evolvement of ideas, especially in online data, suggests data collected in one domain is often not reusable in others. In this work, we develop state-of-the-art NLP models that minimize the need for new annotated data to analyze online text in two settings: detecting implicit gender bias on social media and analyzing emotions expressed in tweets about #BlackLivesMatter protests in 2020.
In the first part, we develop a model to identify systemic differences in social media comments addressed towards men and women by training a model to predict the gender of the addressee and examining which features the model uses to make predictions. The main challenge in this project is preventing the model from focusing on overt predictive features, like names and pronouns, in order to identify subtle features indicative of bias. This approach allows us to identify comments likely to contain bias without needing explicit bias annotations. In the second part, we use a domain-adaptation approach to show that positive emotions like hope and optimism are prevalent in tweets with pro-BlackLivesMatter hashtags and significantly correlated with the presence of on-the-ground protests, whereas anger and disgust are not. These results contrast stereotypical portrayals of protesters as perpetuating anger and outrage. Overall, our work aims to develop NLP models that facilitate text processing in diverse hard-to-annotate settings and provide insights into social-oriented questions.
Bio: Anjalie is a PhD candidate at the Language Technologies Institute at Carnegie Mellon University and a visiting student at the University of Washington, where she is advised by Yulia Tsvtekov. Her work focuses on the intersection of NLP and computational social science, including both developing NLP models that are socially aware and using NLP models to examine social issues like propaganda, stereotypes, and prejudice. She has presented her work in NLP and interdisciplinary conferences, receiving a nomination for best paper at SocInfo 2020, and she is also the recipient of a NSF graduate research fellowship and a Google PhD fellowship. Prior to graduate school, she received her undergraduate degree in computer science, with minors in Latin and ancient Greek, from Princeton University.