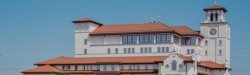
University Calendar
Time of flight and fingerprinting based methods for wireless rogue device detection
Daniel Chege defends his thesis for his MS in Cybersecurity. Watch the presentation on Zoom.
About Daniel
Daniel an alumnus of Maseno University, Kenya did his undergraduate studies with a major in Information Communication Technology and a minor in management. He has worked both in the US and Kenya with an aim to bridge the gap in the IT sector. Whilst in Kenya, he worked as an IT manager providing the much-needed IT services to both marginalized areas and corporate entities. Before pursuing his master’s degree, he worked with a vibrant team at Geonet Communications Group USA providing telecommunication services connecting people from different parts of the world.
In 2019, he enrolled at MSU focusing his interest in computer networks and security. Daniel’s research emphasizes on how device fingerprinting and traffic detection can be used to detect rogue devices and rogue access points
Abstract
This research study examines the extent of IoT devices security breach and unearths threat posed to information security, denial of service, and identity theft. The research exposes how adversaries use IoT devices to masquerade as legit devices by cloning MAC addresses and manipulating SSID. This research examines RSSI viability as a candidate to detect rogue devices at different distances and environments. Results indicates RSSI is effective at certain optimal distance from access point. Furthermore, the study investigates clock skew effectiveness by evaluating how effect size is used to detect rogue access points on the network.
Noble approaches: device fingerprinting and traffic detection are used to detect rogue nodes and rogue access points. Three experiments were conducted with emphasis on the optimal distance, amount of data needed and clock skew offset to detect rogue devices and rogue access points. Additionally, machine learning algorithms are deployed to determine node position while as examine classification performance of data collected from the experiment.
Thesis Committee
- Dr. Christopher Leberknight
- Dr. Boxiang Dong
- Dr. Bharath Samanthula